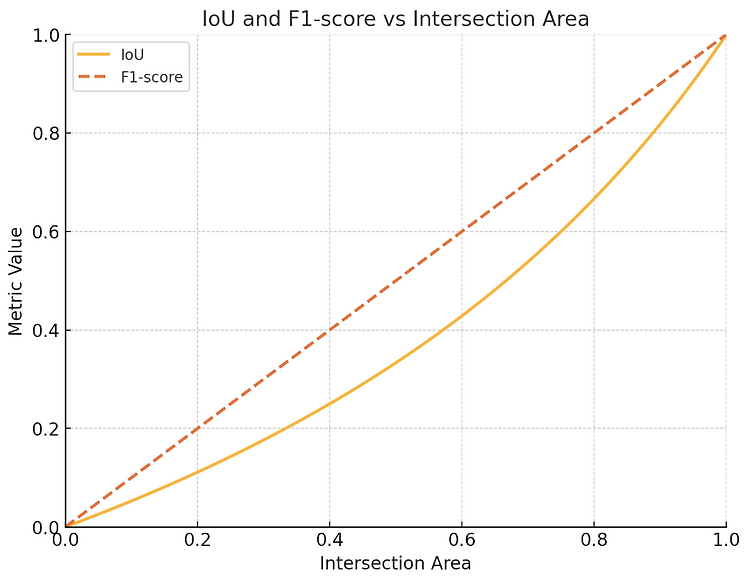
Jaccrad Index(IoU)와 F1/Dice, Coutour Accuracy(F)
·
DL·ML/Study
segmentation task에서 주로 사용하는 metric으로 Jaccard Index(IoU)와 F-score가 있다. 본 글에서는 각각을 이해하고 특징을 살펴본다.Jaccard IndexJaccard index는 [1]에서 처음 정의되어 사용되었으며, Intersection over Union(IoU)로도 불린다. 이는 다음과 같이 정의된다:$$ \frac{TP}{TP+FP+FN}$$ 즉 Jaccard index는 다음과 같이 이해될 수도 있다:$$\frac {A\cap B}{A\cup B}$$ 만약 $A$와 $B$와 완전히 겹쳐져 있으면 1이 나오고, intersect하는 구역이 전혀 없을 경우 0이 나올 것이다. F1 / Dice scoreF1 score는 [2]에서 정의되었으며, 다음과 같..