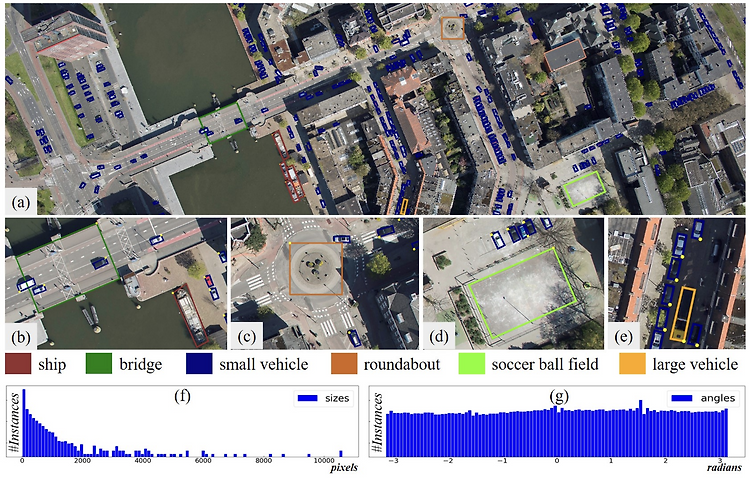
[ODAI] DOTA benchmark
·
DL·ML
Abstract dd Motivation object detection in aerial images(ODAI)는 real-world application에서 많이 사용됨. 하지만, object 크기의 nonuniformity, arbitrary orientation 등은 task를 어렵게 함.(Figure 1 참조) 여러 문제 중 orientation에 대한 문제가 주요 어려움인데, 그 이유는 다음과 같다: rotation-invariant한 feature representation을 만들어야 함. -> 그러나 현재 architecture로는 어려움이 있음. iDeA; 2021년 기준이라 현재에도 계속되는 문제인지는 확인해 보아야 함. horizontal bounding box(HBB)는 oriente..